AI Deep Dive: Understanding Limitations for Better ROI
A Business Owner's Guide to Navigating the AI Landscape
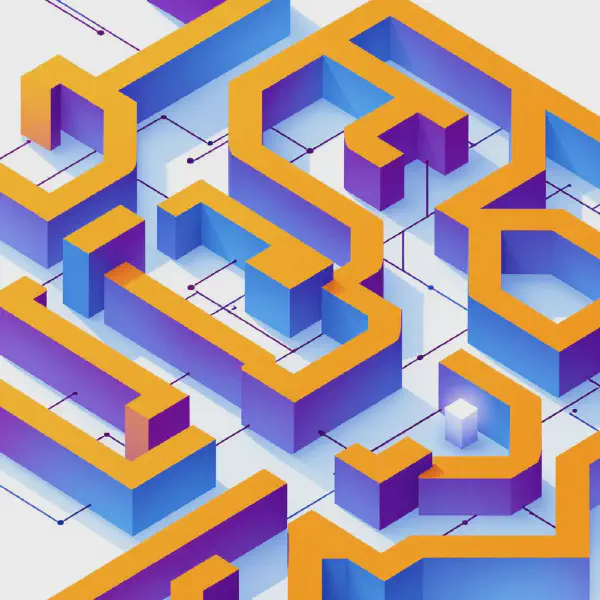
AI ROI
AI has a lot of potential - but businesses struggle to turn this into real ROI that matches expectations.
Want to boost your chances of a successful project? Focus on three things: being realistic about the limits of technology and what AI cannot do, picking the best use case, and assessing honestly if your business is ready for it.
Understanding different types of AI.
AI and Machine Learning are nothing new. Speech recognition, machine vision, and product recommendation engines are just a few examples of tools with proven business applications.
In the last few years, “AI” usually refers to a Large Language Model (like ChatGPT or Gemini) - a type of Generative AI capable of understanding human language and completing various tasks, from research to text generation.
How Do Large Language Models (LLMs) Work?
You type a question in ChatGPT, and it spits out an answer. How?
Human language operates on patterns. See if you can finish a sentence. “It’s raining cats and …”. “Somewhere over a …”
Now take this a step further to a whole sentence. What is the most likely answer to “Hi, how are you?”.
Imagine now that a computer consumed almost all available human knowledge and familiarized itself with patterns - and it has an efficient way to categorize and store acquired knowledge for easy retrieval.
“Training the model” in this case means asking it to figure out how this knowledge should be organized.
Another magic ingredient is context understanding. When you talk to your family about orange juice, mull purchasing an orange jersey and wonder why your orange tree is wilting, the word “orange” means different things. The model needs to learn how to understand the context of the sentence so the correct answer is produced.
The technical “how” is beyond the scope of this article.
Instead, imagine a giant library. You approach the counter with a simple query on how to run a marketing campaign. Your robot helper thinks for a second, disappears up the ladder and returns momentarily with just the right book.
And this is why running AI takes so much power and latest Nvidia chips. To be useful, it needs storage and speed.
Understanding LLM Memory Limitations
In the movie Momento the main character has a damaged long term memory and must leave notes for himself to remember who he is.
LLM does not have a long term memory of your conversations. If you start a new chat, the memory of previous chats is gone. To add new knowledge to LLM you must re-run the training - a process that takes a long time and millions of dollars in power and computing hardware.
But simple question/answer is not very useful when you are trying to talk about your document or brainstorm a new business approach.
The solution is to leave the notes for the model, telling it about the entire conversation every single time. Just like Momento.
This additional knowledge is passed along as a hidden prompt - it’s hidden because you do not see it in your chat transcript. Or it can be pulled from a document (i.e. Claude’s projects and Gemini’s Google Doc). Regardless of how the knowledge is stored and accessed, there is a practical limit to how much of it can be accumulated.
The limit is called a “context window” and is measured in tokens - which is a small part of the word. How small? It depends, but 100 tokens is approximately 75 English words.
Why is it called a context window? As the limit is reached, information acquired at the beginning of the conversation is dropped. In a sense, long term memory is being lost in favor of short term memory.
Obviously, a model with a larger window (i.e. longer memory) is more useful - you can maintain a longer conversation, provide significantly larger documents and not even be aware that the earlier memories are dropped.
Controlling LLM Output - Hallucinations and Bad Answers
When model does pattern match, how do we ensure it doesn’t say something inappropriate, wrong or harmful?
The current solution is to give a model additional guidelines via hidden prompts. For example: be professional, be helpful, always refer to the known data sources.
Sometimes these guidelines may conflict - for example if the model wants to be helpful but also can’t figure out the answer from existing data, it may make something up and present it quite confidently (a behavior referred to as hallucination). Or when being helpful means providing a potentially harmful response.
When such conflict occurs, an output can be unpredictable. Training labs are getting better at enforcing these guidelines and increasing trust and safety, but it is still a fundamental problem - even if hallucinations can be reduced to 3%, it still means sometimes the information is wrong - and wrong unpredictably.
AI does not care if it gives you the wrong answer.
Can AI Reason? Understanding the Decision-Making
The introduction of the reasoning models brings the question - is AI actually intelligent? If they can reason, doesn’t that mean they can make decisions and become more accurate with time?
Think about a problem you had to solve and how you may explain your thought process to others. “Should we offer a refund to this customer? This is their fifth purchase and the issue was caused by a shipping delay. Keeping them happy will lead to further business. Yes, it’s worth offering a refund.”
On a very basic level, the reasoning model follows the same path. First, identify the task, then prepare the plan to execute it and build a checklist to ensure you are successful. Complete each subtask and compare against the checklist.
But AI is not human. It may appear to be “reasoning” or “making decisions,” but it is still a very sophisticated pattern matcher.
AI does not have a concept of common sense. You cannot trust it to make the best decision for your business every single time because it does not have a concept of what the best decision is. In a customer refund example, it may decide to prioritize the short term profitability over the long term brand building.
Considerations for Choosing the Right AI for the Project
There are distinct camps of thought - from “AI will replace everybody” to “AI is useless.”
The truth is somewhere in between - AI is an excellent tool for a specific set of business problems.
We know that the current generation of LLM has a limited memory span, an inability to provide 100% truthful and accurate answers, and non-robust common sense. Until these limitations are addressed, projects that require high stake unassisted decisions, an understanding of a large volume of private information, and a selection of the best and quickest path to the solution may struggle to achieve expected success.
What type of projects may play off this technology’s strengths? Here are just a few examples.
AI Research Agent
Think of this as a better Google news alert. The research agent can search the internet for interesting emerging trends, news relevant to your niche or company, changes in regulations. When an interesting topic is noticed, the agent can analyze the data, create summary, propose new strategic direction or flag potential weaknesses of your business model - and deliver the resulting report to your desk.
Risk level: low. If the conclusion is not helpful or summaries are wrong, you could ignore them.
Helpfulness: high. Done strategically, it can save significant amount of time spotting new trends and opportunities and keeping track of the industry and competitors.
AI Content Agent
Using your strategic document as a guide and research agent as a source of new information, content agent can plan your content calendar, create initial outlines and drafts, or edit and provide feedback on your original content.
Social media and marketing are common use cases, but think bigger - product training manuals personalized to your customers, interactive lead assessment, newsletters.
Risk level: medium. Because the content is meant for your customers, someone should review it first in case of mistakes.
Helpfulness: medium to high. While you do not want to create AI-generated low quality content, it helps smaller businesses to prevent the burnout from doing too much.
AI Voice Agent
This agent can understand the spoken word and be available 24/7 to answer questions about your business, do initial evaluation of leads and schedule appointments. It can be available after hours, filtering support calls.
Risk: medium. This agent directly communicates with the customers without humans in the loop. Additionally some customers may not want to talk to AI.
Helpfulness: high - if risk can be managed - for companies dealing with a large volume of calls.
AI Inventory Agent
Using a vision model, this agent can scan the picture of a new product (or, if sufficient hardware is provided, take an image itself) and register a new SKU, generate tags and create product description. It can interface with existing ERP and use internet to research the appropriate categories - and even measure the item.
Risk: low provided the final description is read and validated by humans Helpfulness: high in environments with low quality/high SKU.
Is your organization ready?
Curious to see what AI automation can do for you? The last ingredient in maximizing AI project success is evaluating your business’s readiness.
Use this assessment to score your operational readiness (and get advice on what your next priorities should be)